
Last year Amazon Prime released a new animated series called “Undone”. TV series that was entirely created through rotoscope animation. You might be familiar with this technique if you’ve watched movies like “A Scanner Darkly” and “Waking Life.” A similar technique was also used in the “Take On Me” video by A-ha. In “Undone”, all the animation was created by Amsterdam-based animation studio “Submarine”. A Team of talented painters and artists from all over the World was engaged to create the final, magnificent effect. What is more, the actual rotoscoping, led by co-producer Craig Staggs, was performed by the super experienced team responsible among others for “A Scanner Darkly”. Nevertheless, the whole process is very time consuming and requires lots of manual labour. What if there would be a technology capable of reducing this handwork by 95%, leaving only the hardest and most interesting parts of it to the artist?
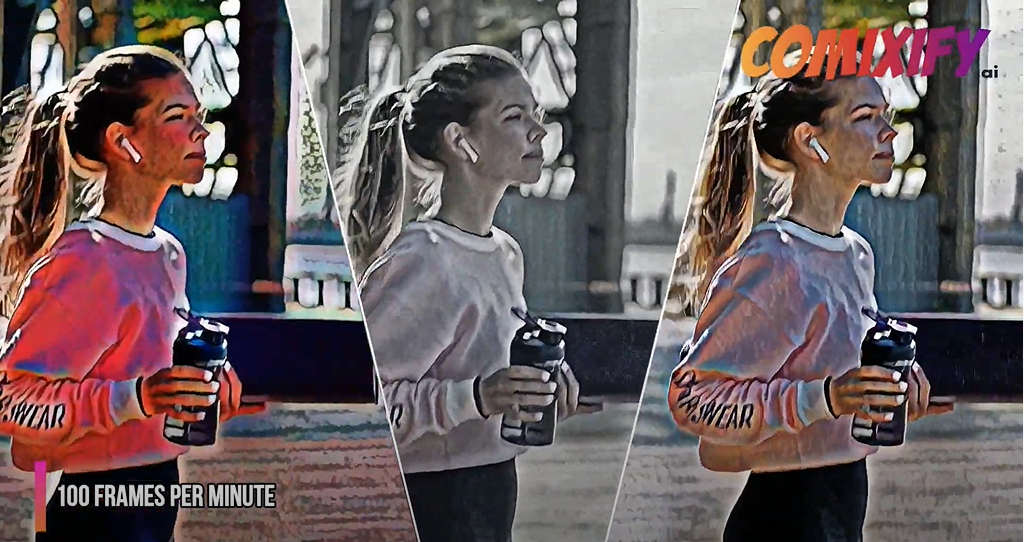
Style Transfer – How we do it
It turns out that such technology already exists, it’s called Style Transfer. Firstly introduced a couple of years ago by L. Gatys et al. in the publication called “A Neural Algorithm of Artistic Style” Style Transfer has come a long way from a very slow optimization based technique to the most recent methods based on state-of-the-art Machine Learning models. In comixify.ai we developed our own proprietary Style Transfer technology based on Deep Learning Generative Adversarial Networks. Our method is able to clone a style of any artist represented by just a few representative painted or drawn samples and then apply it to any video sequence with the outstanding quality. To demonstrate our capabilities let’s dive in one of our styles that we currently work on. We asked an artist to draw a bunch of examples of style inspired by A-ha “Take On Me” music video. Selected samples can be seen below along with original images.
The collection of around 50 pairs of original and corresponding hand-drawn stylized images makes our training dataset. The next step is to train our Machine Learning models to produce similar stylized images automatically, by using our own proprietary Generative Adversarial Network based method. To be more precise, we train two networks Generator (actual stylization model) and Discriminator (subsidiary model that aims to differentiate if a sample is produced by Generated or drawn by an artist). Such a pair of Neural Networks, trained simultaneously, is the core of our training pipeline. Additionally, we also show ground truth samples (prepared by the artist) to the Generator to enhance the training process and make it much faster. Training dataset does not have to be big, usually around 50 images is enough, but obviously the more data the better results will be. To complete the entire process we must add one more thing. Since the main use of our model are videos, we pay special attention to so-called temporal consistency to get rid of visual flickering between consecutive video frames. To address this problem we use special techniques based on optical flow to make our models more robust to such problems. Selected results of described training can be seen below. We demonstrate both chosen pairs of different real and stylized images along with a video sample.
Interested?
If you are interested in creating your own style and apply it to some video sequences to create some beautiful animation then please contact us [email protected] and let’s discuss the details!
Good day! Would you mind if I share your blog with my
myspace group? There’s a lot of people that I think would really appreciate your content.
Please let me know. Many thanks
not at all. Feel free to do so!
It is truly a great and useful piece of info. I am happy that you just shared this helpful information with us.
Please stay us up to date like this. Thanks
for sharing.